Introduction:-
In today’s data‑driven world, choosing the right educational institution can make a significant difference in your career trajectory. The quest for the best data science & analytics institute in Thane is not just about selecting a place to study; it’s about investing in a future filled with opportunities. In this blog post, we explore what makes a great data science institute, discuss essential on‑page SEO factors using practical examples, and provide insights into career‑boosting courses available at an institute that prides itself on excellence and innovation.
Why Rise Institute Stands Out Among Its Peers:-
In the ever-evolving world of data science and analytics, the success of any academic institution depends on its ability to stay ahead of industry trends, offer top-tier mentorship, and foster real-world readiness. Rise Institute has consistently demonstrated excellence in all these areas, establishing itself as a trailblazer in data education.
Cutting-Edge Curriculum Designed for the Future:-
At Rise Institute, the curriculum is not just current—it’s future-focused. Courses are continuously updated to reflect the latest advancements in machine learning, artificial intelligence (AI), big data analytics, and predictive modeling. In addition to these core domains, students gain hands-on proficiency in high-demand tools like Power BI, SQL, Python, and Advanced Excel—essential skills for any aspiring data professional.
By aligning academic content with real-time market demands, Rise ensures its students graduate not only with knowledge, but with the practical toolset and confidence to thrive in today’s data-driven job market.
Learn from the Best: Expert Faculty & Industry Leaders:-
Rise Institute is home to a distinguished faculty composed of seasoned professionals and researchers who bring both academic depth and industry insight into the classroom. One of the standout educators is Ashish Sir, whose dynamic teaching style and deep industry knowledge have inspired countless students to pursue excellence in data science. With years of experience across both academia and real-world analytics projects, Ashish Sir bridges the gap between theory and application like few can. His commitment to mentoring, hands-on training, and making complex topics accessible has made him a favorite among students and a pillar of the Rise learning experience.
Their practical, hands-on approach ensures that students don’t just learn theory—they learn how to apply it effectively in real-world scenarios.
World-Class Infrastructure for Real Learning:-
Students at Rise have access to modern labs, industry-grade software, and a vibrant learning ecosystem. This state-of-the-art environment empowers students to explore, experiment, and excel with the latest data science and analytics tools—preparing them for the demands of a tech-driven world.
Strong Industry Connections with Real Impact:-
Rise Institute maintains close ties with top companies and industry leaders. These relationships translate into guest sessions, workshops, live projects, and internship opportunities that give students firsthand exposure to corporate expectations and practices. It’s this deep industry integration that helps turn classroom learning into career success.
Personalized Mentorship Every Step of the Way:-
Understanding that every learner is different, Rise offers personalized mentorship and career guidance. Whether it’s academic support or career planning, students benefit from one-on-one attention tailored to their goals and challenges—making their journey more impactful and fulfilling.
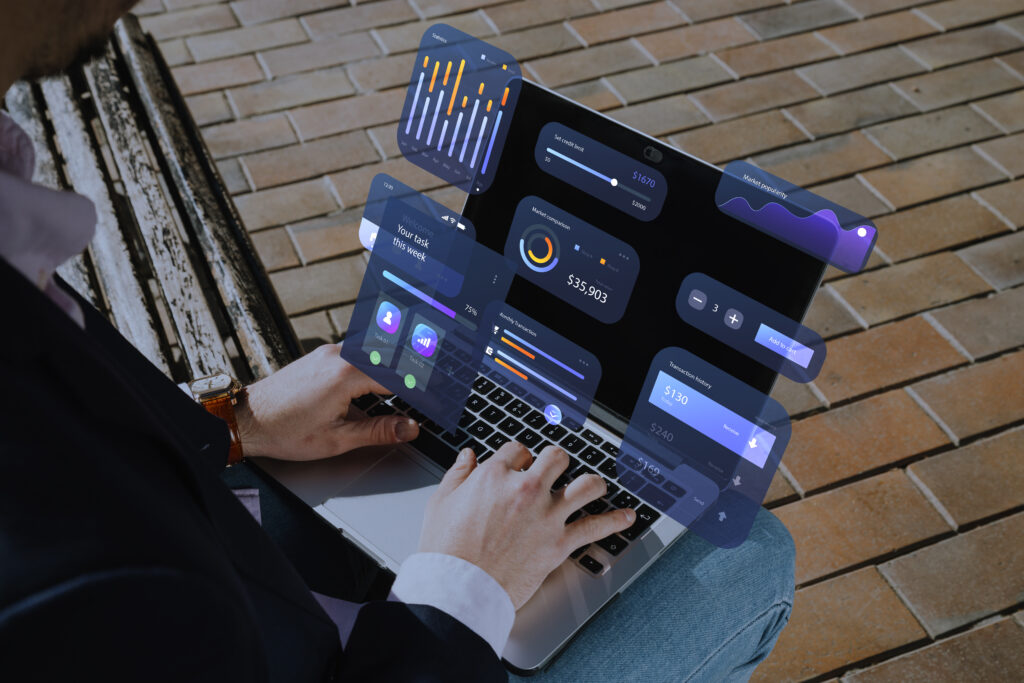
In‑Depth Look at the Curriculum and Course Offerings:-
The robust and diverse curriculum is central to Rise Institute’s success story. It’s designed to take students on a comprehensive learning journey, building a strong foundation while gradually introducing advanced tools and techniques that are vital for today’s data professionals.
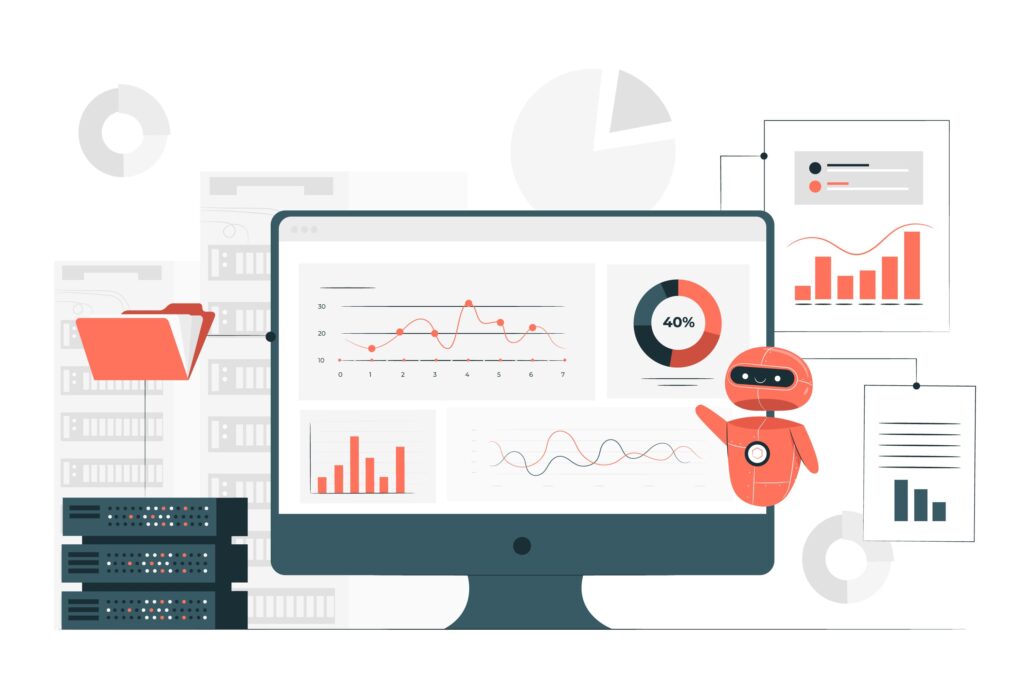
Comprehensive Data Science Courses:-
The data science program is designed to provide a holistic understanding of data analytics, combining theoretical concepts with practical applications. The course starts from the basics of statistics, probability, and programming in languages such as Python and R, and then gradually transitions into more advanced topics:
Data Wrangling and Visualization: Students learn to extract, clean, and visualize data using powerful tools like Pandas, NumPy, and Tableau. Practical examples include creating interactive dashboards and data storytelling.
Machine Learning and AI: The program covers classical algorithms (such as regression, decision trees, and clustering) and advanced machine learning techniques. Hands‑on projects include building recommendation engines and predictive models using frameworks like scikit‑learn and TensorFlow.
Big Data Analytics: Courses on big data introduce students to tools such as Hadoop and Spark, enabling them to process large datasets efficiently. Real‑world projects involve analyzing data from diverse sources to uncover insights that drive business strategies.
Statistical Modeling and Predictive Analytics: The curriculum emphasizes statistical analysis and predictive modeling techniques. Through case studies, students learn to forecast trends and make data‑backed decisions.
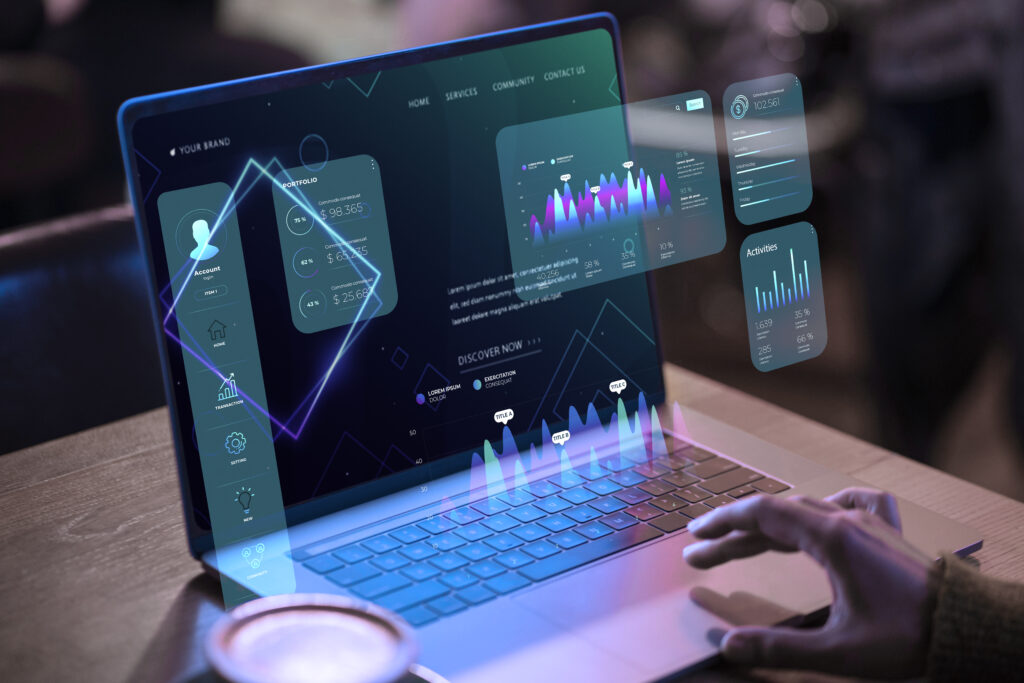
Foundational Components of Data Science: Statistics, Probability, and Programming:-
A cornerstone of a successful data science education is mastering the fundamentals, particularly the mathematics and programming skills necessary for data manipulation and analysis. The early stages of the course are dedicated to deepening understanding in probability theory and statistics—essential for interpreting the randomness and uncertainty inherent in any dataset. In these sessions, students explore probability distributions, hypothesis testing, and statistical significance. An emphasis on rigorous statistical methods paves the way for more advanced analyses later in the program. With a robust grounding in statistics, aspiring data scientists become adept at identifying patterns and relationships within complex datasets.
Equally important is the focus on programming, where languages like Python and R serve as the workhorses of the data science toolkit. Python, revered for its simplicity and versatility, enables students to write efficient code that is both readable and adaptable to a range of applications. Concurrently, R provides a rich ecosystem for statistical computing and graphics, making it ideal for tasks involving detailed data analysis. Through dedicated programming modules, students learn to harness key libraries and packages that streamline data management and visualization. For instance, modules on Python programming cover libraries such as NumPy and Pandas—which facilitate numerical computing and data frame manipulation, respectively—while R sessions emphasize packages like ggplot2 for creating insightful data visualizations.
These courses are designed with hands‑on projects that encourage learners to practice theoretical concepts in a real‑world context, creating a dynamic learning environment that steadily progresses from basic programming constructs to advanced coding techniques. The iterative process of coding, testing, debugging, and refining allows students to build confidence and competence. In doing so, they develop an analytical mindset that is central to the field of data science.
Moreover, these early modules lay the groundwork for more advanced topics by integrating applied mathematics into programming exercises. For example, data science students might begin by exploring linear algebra—a critical component when working with machine learning algorithms—and then progress to more advanced topics such as matrix factorization and gradient descent techniques. Through a carefully curated blend of lectures and interactive lab sessions, learners acquire an appreciation for how theoretical knowledge is applied to practical problem-solving in data science.
Data Wrangling and Visualization:-
One of the most critical aspects of data science is the ability to clean, transform, and visualize data in a manner that reveals hidden insights. The data wrangling and visualization modules of the program focus on teaching students how to extract, cleanse, and reshape raw data into useful information. This process is essential, as the quality of data directly influences the accuracy and reliability of subsequent analyses.
Data Extraction and Cleaning
In the initial phase of data wrangling, students engage with real-world datasets derived from diverse sources—ranging from structured database queries to unstructured data streams from social media platforms. The curriculum emphasizes the importance of data hygiene, guiding learners through methods of handling missing values, dealing with outliers, and resolving data inconsistencies. Tools such as Pandas in Python are introduced as essential instruments that simplify the manipulation of large datasets. With an intuitive API designed specifically for data analysis, Pandas allows data scientists to handle data merging, aggregation, and transformation processes with precision.
Additionally, students learn to leverage R’s powerful data manipulation packages, which are especially useful for statistical analyses. Assignments are designed to simulate scenarios encountered in the industry, where data is often incomplete or noisy. By understanding how to pre-process data effectively, learners are better prepared to ensure that subsequent analytical models are both reliable and robust.
Creating Visual Stories with Data
Visualization is a vital part of data science because it transforms complex datasets into graphical representations that are easier to comprehend and communicate. Visualization techniques not only reveal patterns but also support decision-making by presenting data in an accessible format. In this program, students learn the art and science of data visualization through hands‑on labs that utilize tools like Matplotlib, Seaborn, and Tableau.
Tableau, for instance, serves as a bridge between raw data and actionable insight. With its interactive dashboards, students learn how to design visualizations that communicate trends, correlations, and outliers effectively. The course emphasizes the art of storytelling with data—guiding students on how to craft narratives that resonate with stakeholders. Through a series of practical projects, learners create dashboards that not only depict the data but also tell the story behind the numbers. These projects often involve real-world data, encouraging participants to think critically about both the aesthetics and the informational value of their visualizations.
In addition to these visual tools, the curriculum also delves into advanced visualization techniques, such as network graphs and geospatial mapping, providing a comprehensive overview of how data visualization is integral to data science. Mastering these techniques prepares students to handle the multidimensional aspects of data science projects, ensuring that every insight is visually coherent and analytically sound.
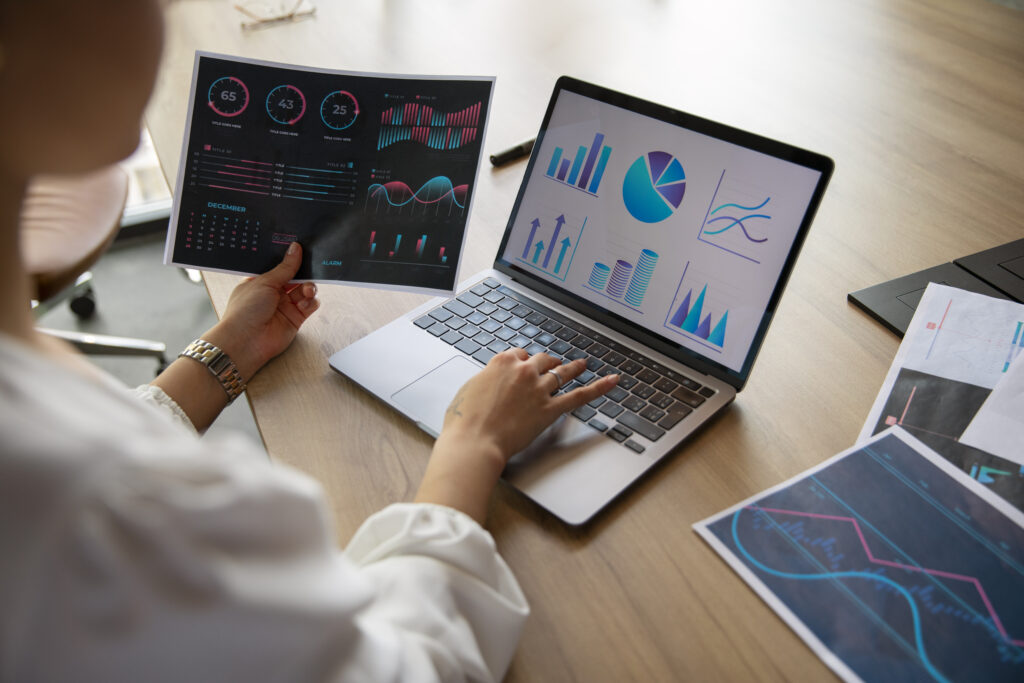
Machine Learning and Artificial Intelligence in Data Science
Once students have built a solid foundation in statistics, probability, and programming, the program transitions into the more specialized and advanced territory of machine learning and artificial intelligence (AI). These domains are at the heart of data science, driving the automation of decision-making processes and enabling predictive analytics that transform how organizations operate.
Classical Machine Learning Algorithms
The journey into machine learning begins with a detailed exploration of classical algorithms that have laid the groundwork for modern data science innovations. Learners are introduced to essential methods such as linear regression, logistic regression, decision trees, and clustering. These algorithms represent the first step in understanding how data models can predict outcomes and uncover hidden patterns in vast data repositories.
For instance, regression techniques allow data scientists to forecast continuous outcomes based on historical data. Decision trees, on the other hand, empower students to visualize decision-making processes, making it easier to segment and analyze data. Clustering methods facilitate grouping data based on inherent similarities, which is particularly useful for customer segmentation and market analysis. Each algorithm is accompanied by hands‑on assignments where students implement these models on real datasets, thereby reinforcing their theoretical knowledge with practical application.
Advanced Machine Learning Techniques
Moving beyond classical methods, the course delves into advanced machine learning and AI techniques that are shaping the future of data science. Students explore ensemble methods, such as random forests and gradient boosting, which combine multiple learning algorithms to achieve better predictive performance. Moreover, the curriculum covers neural networks and deep learning—a field that simulates the human brain’s decision-making process through interconnected layers of nodes. Frameworks such as scikit‑learn, TensorFlow, and Keras are introduced as essential tools for building, training, and deploying these models.
Practical projects in this module often involve designing recommendation engines, building predictive models, and solving classification problems. These real‑world applications serve as the testing ground for theoretical concepts, allowing students to refine their skills through iterative experimentation and model tuning. By applying these methods to diverse datasets—from e-commerce transaction logs to medical imaging—learners gain an appreciation for the breadth and depth of machine learning as a pillar of data science.
The integration of AI into the curriculum illustrates how intelligent systems can enhance decision-making processes. For example, students might work on projects that leverage natural language processing (NLP) to analyze text data, or computer vision techniques to interpret image data. Each project reinforces the notion that, at its core, data science is about turning raw data into intelligence—whether by predicting consumer behavior, detecting fraudulent transactions, or personalizing customer experiences.
Big Data Analytics: Harnessing the Power of Large Datasets
As the volume of data continues to scale, the challenges of processing and analyzing big datasets become ever more critical. This component of the program is devoted to data science applications in big data analytics, where students learn how to manage, process, and derive insights from large-scale data environments.
Tools and Technologies for Big Data
Big data analytics in data science leverages cutting‑edge tools that are capable of handling the vast volumes, velocity, and variety of modern data. Hadoop and Spark, two leading frameworks in the big data space, are central to the curriculum. Hadoop introduces the concept of distributed computing, enabling the storage and processing of data across clusters of computers. By segmenting large datasets into smaller, manageable chunks, Hadoop ensures that even the most voluminous data can be processed efficiently.
In parallel, Apache Spark provides an in‑memory computing framework that dramatically speeds up the processing of large datasets. Spark’s ability to handle iterative algorithms makes it particularly useful in machine learning contexts. Students are trained on these platforms through a series of lab exercises and real‑world projects, where they apply big data techniques to analyze complex datasets gathered from multiple sources such as social media, sensor networks, and enterprise databases.
Applications and Industry Use Cases
The importance of big data analytics cannot be overstated in the field of data science. With businesses increasingly relying on data-driven strategies, the ability to process and analyze big data efficiently is a competitive advantage. Case studies form an integral part of this module, demonstrating how big data analytics has transformed industries such as finance, healthcare, retail, and transportation. Students learn how to design data pipelines that extract valuable insights from data streams, ensuring real-time analytics that inform critical business strategies.
For instance, by harnessing the power of Hadoop and Spark, data science practitioners can develop applications that monitor customer sentiment, detect fraud, and optimize supply chains. These projects are designed to simulate industry challenges, empowering students with the skills needed to handle data at scale. As a result, learners emerge with a comprehensive understanding of big data analytics, well‑equipping them for roles that require both the technical expertise and strategic vision necessary in modern data science environments.
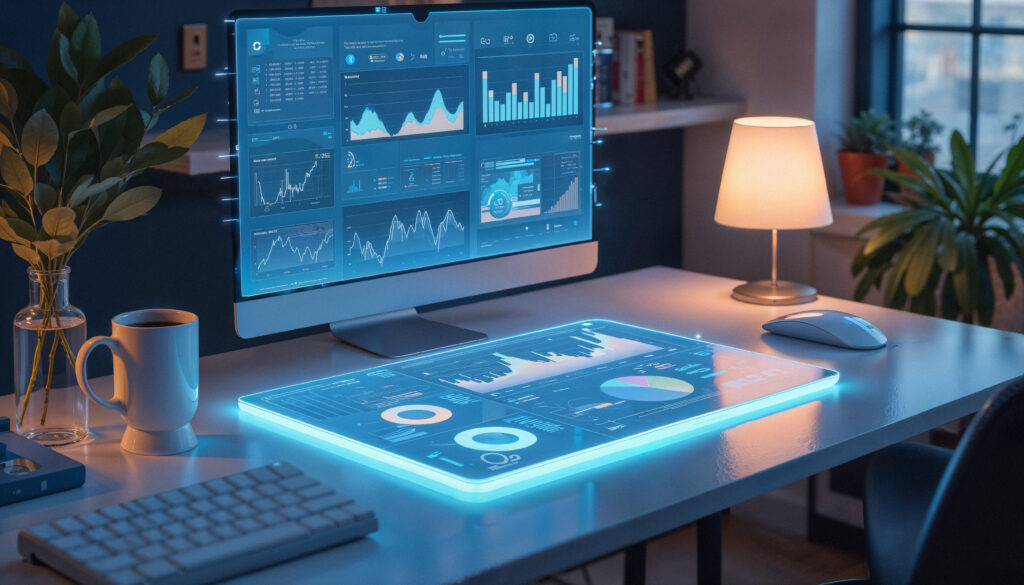
Statistical Modeling and Predictive Analytics
A further critical facet of the data science curriculum is the focus on statistical modeling and predictive analytics. This segment of the course bridges theoretical statistics and applied predictive techniques. The goal is to equip students with methodologies that not only analyze historical data but also forecast future trends with a high degree of accuracy.
Fundamentals of Statistical Modeling
Statistical modeling involves building mathematical representations of real-world phenomena. The program begins by covering linear and nonlinear models, alongside methodologies for parameter estimation and model validation. Using statistical software and programming libraries, students learn how to fit models to data, test hypotheses, and interpret the outcomes. In this context, predictive analytics is introduced as a discipline that relies heavily on robust statistical methods. By grounding their learning in statistical principles, data science practitioners develop the intuition required to select appropriate models and assess their performance objectively.
One of the strengths of the statistical modeling curriculum is its emphasis on case studies. Each case study presents a scenario—ranging from forecasting economic trends to predicting consumer behavior—and requires students to apply their statistical knowledge to derive insights. These exercises hone the ability to discern which models are best suited to different types of data and analytical objectives. The iterative process of model fitting, evaluation, and refinement mirrors the real-world challenges faced by data scientists, making this course component both highly practical and intellectually rigorous.
Predictive Analytics in Practice
Predictive analytics in data science extends beyond the theoretical by integrating machine learning techniques and advanced statistical methods into actionable insights. Students explore predictive modeling projects that encompass forecasting trends, optimizing decision processes, and identifying potential risks before they materialize. In practice, predictive analytics involves an element of uncertainty; hence, a major focus is placed on understanding the limitations and assumptions inherent in statistical models.
Projects in this module are tailored to challenge students with complex, multi-dimensional datasets. These projects require the application of time series analysis, regression modeling, and classification algorithms to make predictions about future trends. In industries such as healthcare, finance, and retail, predictive analytics has proven to be a game-changer by enabling proactive decision-making and strategic planning. Through a series of hands‑on assignments, learners build models that not only predict outcomes but also provide confidence intervals and risk assessments—crucial components for making data‑backed decisions in a real‑world setting.
By blending classical statistical approaches with cutting‑edge machine learning methodologies, the curriculum in predictive analytics ensures that students are well-prepared to address the challenges of tomorrow’s data-driven landscape. This dual emphasis on theory and application embodies the essence of data science—turning data into actionable knowledge and reliable predictions.